Land Use Classification Using
Landsat Data.
Manu Omakupt Valairat
Vunpiyarat Land Development Department Ministry of Agriculture
& cooperative, Thailand
Abstract
Landsat 2 multispectral scanner was used to investigate land use
patterns in the north Thailand. Computer-implemented pattern recognition
techniques were applied for digitally processing and analyzing Landsat 2
multispectral scanner data. Using reformatted digital data obtained by
satellite scanner on 1 February 1977, 15 training areas of known features
in the Chiengmai River Basin were selected. Ground observations on exact
locations for training of the computer were required. Then the spectral
statistics of 15 training areas were carefully examined and correlated
with ground observation data. After the statistical parameters had been
determined, the maximum likelihood algorithm was utilized to classify the
entire data set. Results of the analysis showed that 11 land use
categories in the study area could be classified with a high level of
accuracy. Supervised technique was judged to be the most accuracy for
agricultural mapping in the flat terrain while urban land use mapping was
considered to be sophisheterogeneous nature and continuous nature of the
urban surface cover. However, this study indicated that several level II
land use categories could possibly be identified from satellite data.
Introduction
Since the launching of the first
satellite in July 1972, remote sensing has become acceptable by most
geoscientists for use in identification, mapping, and management of
natural resources. With increasing demand for agricultural products, more
intensive management will be needed to increase yield. The technological
advancements should better meet these needs (Beaubien , 1979). Attention
now turns from conventional aerial photography to small-scale photographs
taken from aircraft at high altitudes or from satellites. Because the
satellites sense reflectances of a given portion of the earth regularly
(at 9 or 18 day intervals) in four bands of the electromagnetic spectrum,
they also provide the necessary information for multitemporal analysis.
Several researches have studied the potential of these MSS data for
natural resources inventories . shimabukuro et al . (1980) studied the
potentiality of computer aided processing of Landsat data to classify tree
species in Brazil and concluded that the automatic classification could be
done effectively in remote regions, where ground and aerial surveys were
often costly. Hoffer (1976) also conducted the computer-Aided analysis of
Skylab Scanner Data for land use, forestry, and water resource mapping and
got a level of classification accuracy.
Mausel et al. (1976)
classified an urban area into its board land-use classes using automatic
data processing (ADP) techniques. The classification results suggested
that all level I and many level II categories of the proposed U.S.G.S.
land-use classification system were possible to identify accurately
through machine-processing of Landsat multispectral data. Forster (1980)
also mapped urban residential cover using Landsat digital data.
Much attention has been given to the identification and mapping of
agricultural lands (which et al. 1979). He conducted that manual
interpretation of Landsat images permitted the rapid construction of
regional map products.
Objectives
The objectives of
this study are as follows:
- To identify and map Chiengmai river basin into broad land use
categories by digital analysis of Landsat MSS data.
- To evaluate the classification performance and obtain a quantitative
evaluation.
The Study Area
The Chiengmai *River Basin
located in the Chiengmai, northern Thailand area was selected for
study. The study area, is located between 18° 45'Nand 99ΊE. The
climate of the area is Tropical Monsoon with moist summer and dry
winter. The average annual temperature is about 25.28ΊC and mean
annual rainfall 121.9 cm. The Ping River flow through the study area
from north to south is the longest river in Chiengmai province and
also in the nation. Bounded by the forest-covered Dan Lac Ranges, the
study area occupies an area of 732 sq kilometre with an elevation
reaching 308.1 metre. Within this area, most of the agricultural
activities are found. Only three major land uses are present
(Cropland, urban, and forest), but the combinations and percentages
vary considerably in identifying land use patterns. Most soils,
commonly found under irrigation, are poorly drained alluvial soils
yielding an average of 3,075 kg/hectare of rice production. This area
tends to be one of the most important vegetable producing area in the
northern Thailand (figure 1)
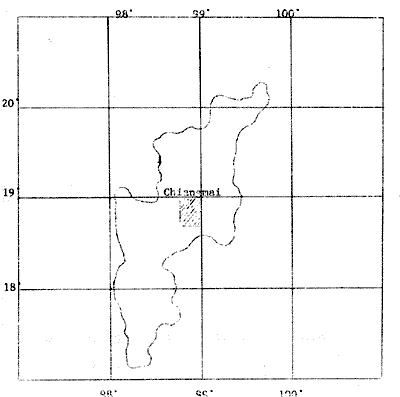
Locatio of the Study
Area.
Method
Landsat scene ID
No. D-2741-02510 acquired on February 1, 1977 was utilized for the
study. Black and white imagery of MSS-5 and MSS-7 bands were printed
at 1:500,000 scale for the test site selection. Landsat computer
compatible tape (CCT) was subjected to be reformatted to produce an
alphanumeric image of the study area. The grey scale images, produced
for each of the four bands of MSS data, were then used in the
drlrvyion og training areas , The RECOGX program, modified from the
RECOG package of Co loralo State University by the Asian Institute of
Technology (AIT), was used to carry out a supervesed classification
for the entire study area. In the first stage, the locations of 26
known earth surface features, resulting in a total number of 15
training classes or areas, were designated to the computer system. A
statistic processor containing in the program eas then requested.
Consequently, a statistic processor provided a set of statistical
parameters for each group of training areas. These parameters included
the class mean, standard deviation, histograms, correlation matrix,
spectral plots, and coincident spectral plots.
In order to
judge these spectral values of 15 training areas, field checking was
made in February 1980 to most of the areas accessible by road. In
addition land use maps, previously made in 1977 from conventional
aerial-photography interpretation, at 1:50,000 scale were also
prepared so that all inaccessible training fields could be accurately
located. Then the spectral statistics of 15 training classes were
carefully examined and correlated with ground observation data. Taking
into account the statistical parameters, 4 classes were considered to
be less reliable . the remaining II classes were input to a Caussian
maximum likelihood classifier which was used to classify all manually
selected training areas and test sitd Computer classification results
could be displayed in a maplike format or tabular output formats.
Results and Discussion
In the classification
sequerce, each resolution element was assogmed to one of the spectral
classes defined during the development of the training statistics.
Based on the supervised classification results, a description of each
of these II land use classes was presented below.
Figute IV Reflectance values for a major land use classes for all
band combinations. FOD1 Deciduous forests
mostly dominated the Dan Lao Range along the west side of the study
area. This class was considered as full canopy closure deciduous
forest. FOD2 Partial canopy closure mixed deciduous
forest FOC1 Full canopy closure mixed deciduous forest, exposed
to sunlight. FOC2 Full canopy closure dry dipterocarps OCHD
Mixed stands, predominantly dry dipterocarps or brush, with mature
orchards and trees. RFI1 seedling to-young rice of field crops
including tobacco , peanut, soybean, mung bean corn garlic, and onion
in irrigated rice fields. RFI2 Young rice or field crops
including tobacco, peanut, soybean, mung bean, garlic, and onion in
irrigated rice fields. RFN1 Non-irrigated, young to semi-mature
field crops RFNS Non-irrigated, rice stubble or grass UBP 1
Transportation, communication, utilities UBP 2 Suburban,
other
Summary of spectral statistic of these II land
use classes was shown in Table 1. The supervised classification
allowed us generally to distinguish forest, crop, and urban land.
Spectral reflectance curves derived from the training data statistics
for the four major land use classes indicated good separability of
land cover and land use classes (Figure 2). Because a right portion of
the Chiengmai River Basin is relatively flat, with a fairly simple
vegetation cover, this no doubt contributes to the success of the
classification.
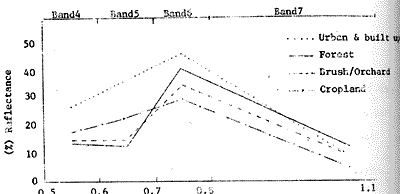
From Table
1 the spectral statistics revealed that identification of forest types
was quite possible. Those were 3 subclasses of mixed deciduous forest
and 2 subclasses of dry dipterocarp or deciduous dipterocarp
represented FOD 1, FOD 2, FOC 1, FOC 2 OCHD , respectively. To obtain
the classification accuracy, the percentages of correct classification
were calculated by using the following formula

Thus, the
overall accuracy classification of forest subclasses was between 85
and 100 percent, depending on the complexity of the area (Table 2).
Areas with more homogeneous forest cover exhibited high classification
accuracy. Apart from type differences, the major factors contributing
to misclassification were density, soil moisture, high relief, and
exposure of slopes. Areas exposed to sunlight (FOC 1) and contained
dense canopy closure (FOD 1) and a higher reflectance than those with
partial canopy closure and their associated shadow areas (FOD 2). The
highest percentage of correct classification was obtained from the
class FOC 1 (100 percent). This could be explained by the fact that
class FOC 1 being trained to the computer was quite small and
sufficiently homogeneous. For the area showed up with more variation
(OCHD) the accuracy was lower than all the above-mentioned forest
classes. However, the results showed that a supervised classification
is proved to yield a very good results although some authors,
including Beaubien (1979), and Hoffer (1976), claimed to achieve
better results with an unsupervised classification.
Like the
forest class, the classification accuracy of cropland class could be
obtained in the same manner (Table 2). The first three subclasses were
distinguished and characterized by irrigated water in the areas. Based
on crop calendars, rice and some field crops were grown in these
irrigated lands (Figure 3). Since most irrigated lands occurred in the
study areas , the contrast between irrigated cropland and surrounding
dry land was great the principal aids to identification of crops
included crop calendars and reflectance differences between crops.
Figure 3 indicated that identification of specific crops was
handicapped by the overlap in the growing seasons. Because fields were
still in early stages of growth with the exposed underlying water, the
reflectance curve of cropland differed apparently from typically
normal curve.
The fourth field (RFNS) was grass and recently
cutting crops with the exposed underlying soil modifying the normal
reflectance curve for green vegetation. Variations from typically
spectral curve caused decision making on green vegetation class to be
difficult. The benefit of ratioing could help the authors to make such
decision. So the reflectance values for major land use classes were
graphed in Figure 4 showing all possible two dementional combinations
of the four bands. The quite high correlation of the two visible bands
an the two infrared bands was clearly evident. Theoretically, the
green vegetation would give a visible/IR reflectance ratio value of
less than 1.0. This could be explained that the underlying water had a
mare pronounced effect on the spectral values of vegetation class were
considered to be density, stage of growth, flooding, drainage
patterns, and locations.
Finally the urban and built-up land
was sub divided into 2 subclasses, namely UBP1 and UBP2 the class UBP1
was predominantly a mixture of airfield (concrete), roof tops, and
green vegetation. The combined spectral response from the various
proportions of all phenomena permitted the differentiation of one
urban class from another. The UBP2 class was primarily a mixture of
rather highly reflective materials i.e. concrete and dry bare soil.
Urban residential area typically exhibited heterogeneous nature of the
urban surface cover. Another related problem which limited more
detailed classification was the continuous nature of the cover
classes. In UBP1 class residential density was high with few
established large trees, another had similar density with no
vegetation. Between each of these, intermediate example occurred
(Forster, 1980). Thus the classification accuracy of urban classes
ranged from 66.7 to 8.9%.
Conclusion
Analysis
of Landsat MSS data from the February 1,1999 shows that the supervised
technique can be used effectively to distinguished cropland, urban,
and forest land. The overall classification accuracy is 93. 2%
Classification of cropland proves feasible through the use of computer
assisted classification procedure conducted with the aid of crop
calendars and reflectance spectrum. Four crop categories can be
identified. Crop type is detectable with difficulty owing to the
confusion of irrigated water presented in the fields.
This
study shows that data obtained by Landsat can provide information
needed for a general mapping of forest and urban land. Mapping of the
level II category is accurate but not always immediately discernable.
The production of land-use maps has become a relatively simple matter
with the recent wide availability of satellite data. Present satellite
data are useful primarily for level I mapping.
References
cited
- Beaubien, J., 1979. Forest Type Mapping from Landsat Digital
Data, Photogrametric Engineering & Remote Sensing,
45:1135-1144.
- Feasibility Report 1970. Kud Reservoir Project. Royal Irrigation
Department, Bangkok, Thailand.
- Forster, PB.C. , 1980. Urban Residential Ground Cover Using
Landsat Digital Data, Photogrammetric Engineering and Remote
Sensing, 45:547-558.
- Hoffer, R.M., 1976. Computer-Aided Analysis of Skylab Scanner
Data for Land Use Mapping, Forestry, and Water Resources
Applications. LARS Information Note 060176, Purdue University , West
Lafayette, Indiana.
- Hoffer, R.M., 1976. Techniques and Application for
Computer-Aided Analysis of Multispectral Scanner Data, LARS
Information Note 062276, Purdue University, West Lafayette,
Indiana.
- .Mausel, P.W., W.J. Todd, and M.E. Baumgardnar, 1976. An
Analysis of Metropolitan Land-Use by Machine Processing of Earth
Resources Technology, Satellite Data, LARS Information Note 031275
Purdue University, West Lafayette, Indiana.
- Shimabukuro, Y.E., P. Hernadez, Filho N.F. Kotter and S.C. Chen,
1980. Automatic classification of Reforested Fine and Eucalyptus
Using Landsat Data, Photogrammetric Engineering and Remote Sensing,
46:209-216.
- Welch, R., H. C.Lo, C.W. Pannell, 1979, Mapping chinas New
Agricultural Lands, Photogrammetric Engineering and Remote Sensing,
45:1211-1228.
|