Case studies for landcover
chance analysis using Micro-Asean
Abd-alla Gad A.
G. National Research Center & Remote Sensing Center,Cairo,
Egypt
hoji Takeuchi,Kauhei Cho,Mitsunori Yoshiura
Remote Sensing Technology Center of Japan, Tokyo 106, Japan
Abstract MICRO – ASEAN, the image analysis
software for a personal computer developed through the join research
project between ASEAN countries and Japan, has now become available for
various studies for monitoring of landcover change using remote sensing.
Because of its high cost performance and easy-handling, MICRO-ASEAN has
been used as one of the core systems for the training courses for remote
sensing data analysis. This paper reports some results obtained from the
case studies conducted using MICRO-ASEAN at the training course as well as
at the joint research. Two kinds of case studies are reported, one is the
analysis using MOS-1/MESSR and Landat/MSS for landcover change at Tama
Area, one of new urbanized areas in Tokya Capital Region, which was
conducted as the subject for the remote sensing training course. The other
is the case study for monitoring of the wide-range landcover condition is
Thailand using NOAA/AVHRR data combined with MOS-1/MESSR data. Through
these case studies, MICRO-ASEAN was proved to be used effectively and
practically for landcover monitoring by remote sensing.
Introduction Since a personal computer technology has
made a remarkable progress, the image analysis software using a personal
computer for practical use of remote sensing data has become possible to
be realized. MICRO-ASEAN (MICRO computer-based Advanced System for
Environmental Analysis with remote sensing data) was developed on the
above background through the joint research project with ASEAN countries
by Special Coordination Funds of the Science and Technology Agency of
Japan. This software was developed based on the software package called
MICRO-TIPE developed by Tokai University and extended to the system
including the functions for GIS and the analysis of multi-temporal and
multi-stage remote sensing data.
MICRO-ASEAN has now become one of
the important systems used for the international cooperation for promoting
remote sensing data utilization in developing countries, such as the
training course for remote sensing technology. This paper reports some
examples of the case study using MICRO-ASEAN, which will be very useful as
the subject for remote sensing data analysis at the training courses as a
typical study for monitoring of landcover change. Two kinds of case
studies are reported, one is the analysis using MOS-1/MESSR and
Landsat/MSS at Tama Area, one of new urbanized areas in Tokyo Capital
Region, which was conducted as the subject at the training course held by
JICA (Japan International Cooperation Agency). The other is the case study
for monitoring of wide-range landcover condition in Thailand using
NOAA/AVHRR data combined with MOS-1/MESSR data, which was conducted as a
part of the joint research described above.
Landcover Change
Extraction of Tama Area Using MESSR and MSS Temporal Images
- Background and Location of Study Area
The increase of the
population in Tokyo Capital Region has urged the society to extend its
residential area. This trend has an impact on the environment. The
purpose of this study is to test the applicability of MICRO-ASEAN system
for detecting the change of landcover through a particular case. Two
satellite images for the study area were used; MOS-1/MESSR data Nov.
1990 and Landsat/MSS dated Oct. 1981. The test site “Tama Area” is
located 30-40 km from the center of Tokyo on the Tama Hill to the west.
A new planned city “Tama New Town” has been developed in this area. The
total area of the New Town is about 3,016 hectares. Development of Urban
facilities such as roads, water works, etc. is carried out for a planned
population of 370,000 people, while housing constructing is planned for
an expected residential population of 310,000.
- Methods
The data analysis flow is shown in Fig. 1. Two
approaches were applied to investigate both of landcover condition and
landcover change. The first approach as landcover classification
procedures by supervised maximum likelihood classification method. The
second one was the change detection using normalized vegetation index
(NVI).
In the second approach, a normalization procedure was
applied for both of MESSR and MSS images to follow the change in NVI.
Then, the discrimination of vegetated and non-vegetated land was
performed for both temporal images. Landcover change between vegetation
and non-vegetation was extracted using the result of discrimination by
NVI.
- Results and Discussions
The system allowed to select 14
landcover categories including total 30 training areas. It was possible
to confirm a particular spectral signature of each object. The result of
confusion matrix of the training data set showed a significant
correlation in classification of the following categories; Factories
(85.8%), Forest 1 (84.8%), forest 2(92.3%), Bare soil (93.9%), Water
(97.6%), City 4(81.%), City 1(88.5%) and Graves (84.9%). However, weak
coincidence were found in other different categories (e.g. Golf Courses
and Secondary Forest). This can be attributed to the mixing of these
categories in feature space and also to the seasonal effect of
vegetation cover. The result of landcover classification of Tama
Area is represented by the color-coded image shown in Fig. 2. The result
of the area measurement of classified data is shown in Table 1. The result
shows an emphasis on creating new urban areas. The old residential areas
(City 1 and City 2) represent 6.7 and 2.9% respectively of the total area.
However, less dense built-up areas record higher percentage of the total
study area (i.e. City 3=19.6% and City 4 =15.5%). It is also remarkable to
notice a significant wide coverage by forested area (20.3% total area of
Forest1, Forest2 and Secondary Forest).
Fig. 3. shows the result
of change detection using NVI. This image was obtained though the
filtering of resultant image by change detection procedures between
vegetation and non-vegetation. Table 2. shows the result of area
measurement of three kinds of changing patterns, 0;no change, 1;change
from vegetation. The result shows a significant change from vegetation to
vegetation. The result shows a significant change from vegetation to
non-vegetation (type-1). By comparing Fig. 2 with Fig.3, the changed area
of type-1 almost corresponds to the surrounding urban areas of Secondary
Forest. This suggests that significant change from the secondary forest to
urban occurred during 9 years difference between the observation dates of
MESSR and MSS images.
The NVI index used in this case study is
considered to be a satisfactory indication of vegetation coverage and to
be used effectively in detecting the change in landcover by using
multi-temporal images. Also this method seems to give more reliable result
for the change detection than the method by performing the landcover
classification of dual images using maximum likelihood method (MLM),
because the landcover classification procedure is more simple for the
method using NVI than that by MLM.
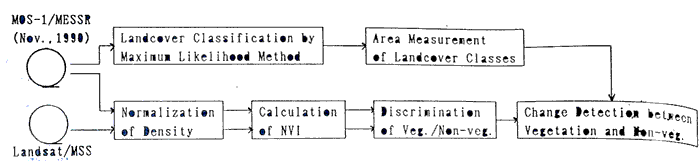 Fig. 1 Data analysis flow for landcover
change investigation in Tama Area
Table 1 Result of
area measurement of landcover classes in Tama Area
Code |
Landcover Class |
Area (km) |
Occupation Rate (%) |
Code |
Landcover Class |
Area (km2) |
Occupation Rate (%) |
1. |
Factory |
25.9 |
5.06 |
8 |
Secondary Forest |
52.4 |
10.23 |
2. |
Agricultural F. |
69.5 |
1357 |
9 |
Water |
1.6 |
0.31 |
3. |
Rice Field |
48.6 |
9.50 |
10 |
City4 |
79.5 |
15.53 |
4. |
Forest1 |
13.5 |
2.63 |
11 |
City1 |
34.2 |
6.69 |
5. |
Forest2 |
17.3 |
3.38 |
12 |
City2 |
15.1 |
2.94 |
6. |
Bare Soil |
12.1 |
2.36 |
13 |
Graveyards |
4.4 |
0.85 |
7. |
Golf C. (Grass) |
37.3 |
7.28 |
14 |
City3 |
100.5 |
19.63 | Table 2 Result of area
measurement of change patterns between vegetation and non-vegetation.
Code |
Change Pattern |
Area in Km2 |
Occupation Rate (%) |
0 |
No change (both of Vegetation and Non-veg.) |
462.1 |
90.26 |
1. |
Change from Vegetation to Non-vegetation |
39.1 |
7.63 |
2. |
Change from Non-vegetation to Vegetation |
10.8 |
2.11 |
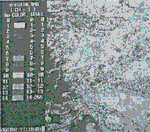 Fig 2. Landcover classification image of Tama
Area |
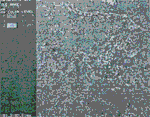 Fig 3. Result of change detection of Tama
Area | Landcover
Change Extraction in the Central Plain of Thailand Using NOAA/AVHRR
Combined with MOS-1/MESSR.
- Background and Objective
The analysis of multi-temporal
images described in 2.1 is considered to be the basic approach to
monitor the landcover condition by remote sensing. However, t is very
difficult to monitor the wide-range are using only Landsat or
MOS-1/MESSR images because of their, which covers narrow coverage. On
the other hand, NOAA/AVHRR image, which covers over 2,000 km swath, is
considered to be the most suitable data for wide-range monitoring. In
this case study, the analysis of NOAA/AVHRR image combined with
MOS-1/MESSR image was tried to monitor the landcover change of the whole
area of the central plain of Thailand. The combined use of the sensors
which have different resolution each other is expected to enable the
quantitative analysis on the special landcover type such as vegetation
converge in wide-range areas.
- Methods
The data analysis flow is shown in Fig. 4. The
important point of this approach is to obtain the relation between the
spectra of AVHRR data and the occupation rate of a special landcover
type within a pixel size of AVHRR through the regression analysis of
AVHRR and MESSR data. The occupation rate of the landcover can be easily
compted from the result of the classification of the landcover type from
MESSR image. The test site where MESSR data are superimposed onto AVHRR
is limited in a narrow area, however, the relation obtained from the
test site can be extended to the area of AVHRR coverage and the
quantitative information on the distribution of the landcover type can
be estimated in wide-range areas.
- Results and Discussions
The test site for AVHRR-MESSR
superimposition was selected in the north-east area from Bangkok of the
Central Plain. Three types of landcover, vegetation, dry bar soil and
wet bare soil, were extracted from two temporal MESSR images (taken on
Dec. 7, 1989 and on Jan. 11, 1990). The extraction of these landcover
types were performed using the normalized vegetation index (NVI) for
vegetation and the brightness feature (BRT) for dry and wet bare soil.
The latter feature was obtained by the summation of all band data of
MESSR. Table 3 shows the result of the regression analysis using the
percent occupation of the three landcover classes obtained from MESSR
the spectral features of AVHRR data. Three types of spectral features
were used for AVHRR, NVI, BRT (summation of the first and second bands
of AVHRR) and CH4 (the fourth band of AVHRR, the thermal land). In Table
3, a significant high correlation is found between vegetation occupation
rate and NVI feature of AVHRR. For the occupation rate of dry bare soil,
the multiple regression using NVI and CH4 of AVHRR gives relatively high
correlation. For wet bare soil, the result is not satisfactory, which is
considered to be due to small occupation of wet bare soil in the test
site. Fig. 5 shows the results of estimation of the occupation
rate for vegetation and dry bare soil in whole area of the Central Plain
using the results in Table 3. The NOAA/AVHRR images were generated by
superimposition of multi-data images around the date of MESSR observation.
This procedure can offer the image in which some cloud covers are
eliminated. The result indicates that a significant change of vegetation
cover occurred over the wide areas in the Central Plain curing only one
month at the beginning of dry season. It is also found that the
significant change of the coverage of dry bare soil occurs, which
corresponds complementary to the change of vegetation cover. This suggests
that there are some problems on the soil conditions in this test site.
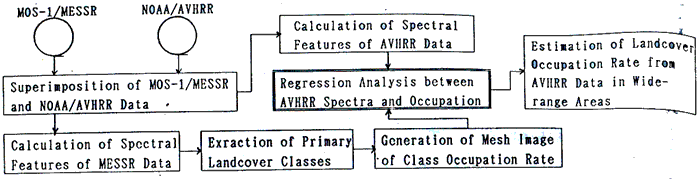 Fig. 4. Data analysis flow for
wide-rage monitoring using NOAA/AVHRR combined with MOS-1/MESSR.
Table 3 Result of regression analysis between AVHRR
spectral features and occupation rate of primary landcover classes.
Target Variables |
Data |
Observation Variables amp; Correlation
Coefficient |
Percent Occupation of Landcover Class |
Single Regression |
Multiple Regression |
NVI BRT CII4 |
NVI+CII4 BRT+CH4 VI+BRT+CII4 |
1. Vegetation |
89/12 90/01 |
0.845 0.625 0.918 0.837 |
0.845 0.846 0.921 0.930 |
2. Dry Bare Soil |
89/12 90/01 |
0.699 0.481 0.343 0.558 |
0.822 0.825 0.731 0.774 |
3. Wet Bare Soil |
89/12 90/01 |
0.627 0.038 0.399 0.020 |
0.632 0.646 0.404
0.429 | Conclusions Through
two case studies described above, MICRO-ASEAN was proved to be used
effectively to investigate the landcover change as well as the present
landcover conditions. For the investigation in a small local area
exemplified in the first case study, the image analysis using
multi-temporal images such as Landsat/MSS or TM and MOS-1/MESSR seems to
be satisfactory to achieve the extraction of landcover change. Also the
method for change detection in the first case study seems to be able to
become a standard procedure for the practice of the detection of landcover
change because of its simplicity and easiness for landcover classification
procedure. The second case study is aimed to establish the
procedure for achieving the monitoring of the Wide-range landcover change.
Especially, the combination of different type of sensors is considered to
be an very effective method for the practical applications and to be the
important trend as the monitoring techniques using remote sensing data.
MICRO-ASEAN seems to be able to be used successfully from now on
as the standard system for the international cooperation through the
practice for the case studies on landcover monitoring described in this
paper. Finally, the second case study described in this paper was
conducted through special Coordination Funds of the Science and Technology
Agency of Japan in fiscal 1990. References
- Takeuchi S., K. Cho, M. Yoshimura et al, 1991 ; Development of Image
Analysis Using Multi Data Source for Monitoring of Tropical Area,
Technical Report of Joint Research on the Enhancement and Application of
the Remote Sensing Technology with ASEAN Countries Prepared for the
Symposium on Join Research project on the Enhancement and Application of
the Remote Sensing Technology between ASEAN Countries and Japan, Feb.
5-7, 1991, Bangkok, Thailand.
- Fukue K., H. Shimoda, and T. Sakata, 1986; Development of A Personal
Computer Based Image Processing System, The 7th Asian Conference on
Remote Sensing, Seoul, Korea.
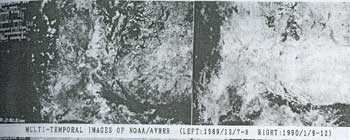
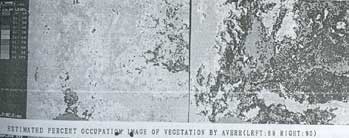
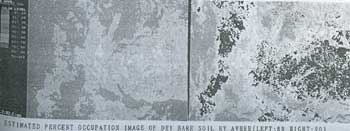 Fig. 5. Result of the estimation of the
occupation rate or primary landcover classes using the result of
regression in Table 3 and NOAA/AVHRR images in the Central Plain of
Thailand.
|